Submitted by Kim Spring, Business Analyst
Driving is an integral aspect of modern life, serving as the primary means of mobility for millions worldwide. However, with the increasing number of vehicles on the road, the risk of accidents and traffic-related injuries continues to be a significant concern.
Fortunately, predictive risk analytics are the up-and-coming game-changer for improving road safety and helping both drivers and insurance companies better assess and mitigate potential risks.
What are Predictive Risk Analytics?
Predictive risk analytics is the use of advanced data analysis techniques, machine learning algorithms, and historical data to forecast potential risks. In the context of driving, it involves predicting the likelihood of an accident or incident based on factors like a driver’s behavior, road conditions, vehicle health, weather patterns, and more. By analyzing these data points, predictive models can assess risk in real time and offer insights into how a driver’s actions may lead to dangerous situations.
Probably the best known “old school” example of of this is why UPS trucks began avoiding left hand turns in the 1970’s. According to GE.com, ” UPS have designed their vehicle routing software to eliminate as many left-hand turns as possible… As a result, the company claims it uses 10m gallons less fuel, emits 20,000 tons less carbon dioxide and delivers 350,000 more packages every year.”
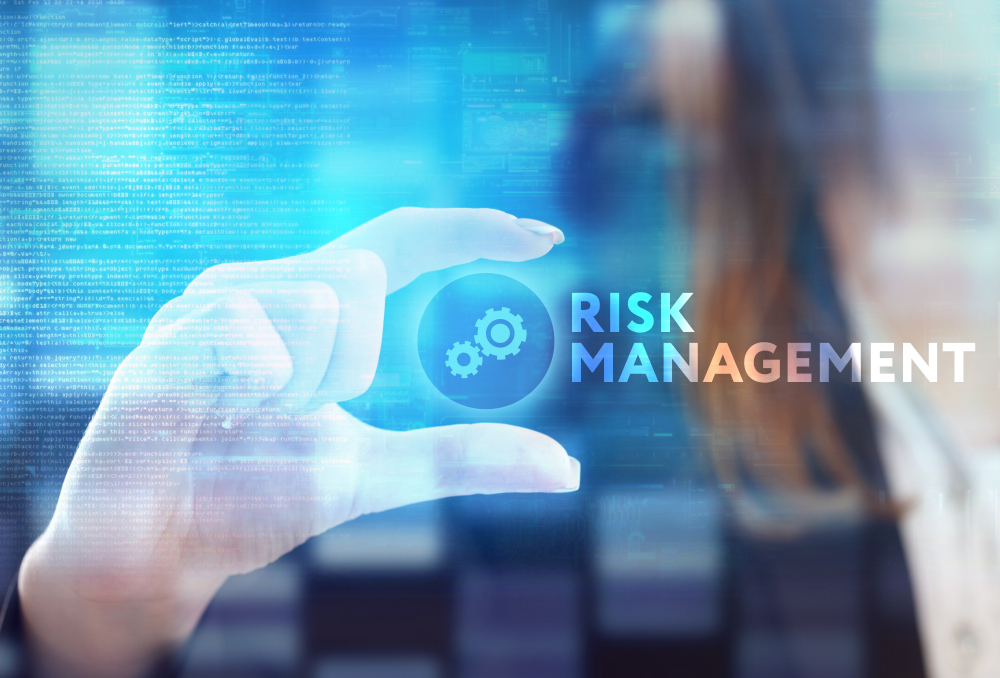
How Does It Work?
At its core, predictive risk analytics for drivers relies on data collected from various sources. This data is then processed through machine learning models that can identify patterns and correlations. These patterns help determine the level of risk a driver may face at any given moment.
Some key data points that are commonly analyzed include:
• Driver Behavior
Speeding, hard braking, sudden lane changes, and erratic acceleration are signs of risky driving behavior that predictive analytics can track.
• Vehicle Data
The condition of the vehicle, including maintenance history and engine diagnostics, can provide insight into potential breakdowns or accidents.
• Traffic and Weather Conditions
Data on weather conditions, road quality, traffic congestion, and even time of day can influence accident risk. Predictive models can use this information to assess external threats.
• Driver Demographics
Age, driving experience, and driving history are all factors that predictive analytics can use to assess individual risk.
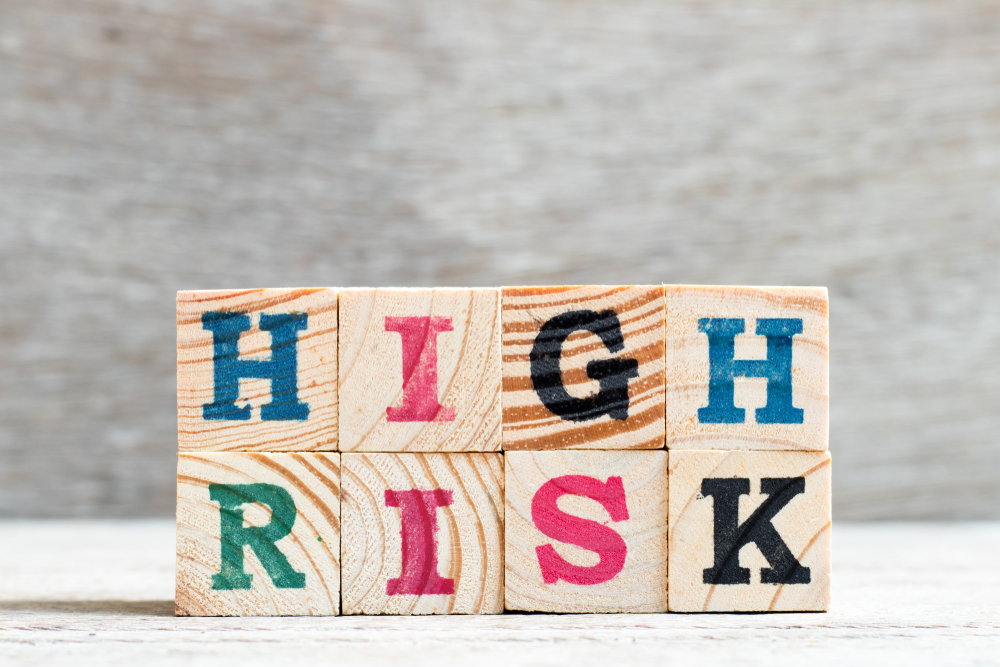
Applications for Predictive Risk Analytics for Drivers
Insurance Industry
One of the most significant applications of predictive risk analytics is in the insurance industry. Insurance companies use these models to assess risk profiles of individual drivers and calculate premiums. By leveraging predictive analytics, insurers can offer usage-based insurance (UBI) where premiums are based on a driver’s actual behavior rather than general demographics, rewarding safe driving with lower rates and encouraging more responsible road habits.
Driver Assistance Systems
Many modern vehicles are equipped with advanced driver assistance systems (ADAS), which are designed to reduce human error and improve safety. Predictive analytics can enhance these systems by providing real-time data on a driver’s habits and the current road conditions. This allows for more responsive safety features such as automatic braking, lane-keeping assistance, and collision warnings.
Fleet Management
For businesses that operate fleets of vehicles, predictive risk analytics are invaluable. Fleet managers can use this technology to monitor driver behavior, track vehicle maintenance, and predict when a vehicle might be at risk for a breakdown or accident. Using these predictive insights, companies can make informed decisions on how to improve driver safety and optimize routes.
Road Safety Improvements
Governments and municipalities can use predictive analytics to identify areas with higher accident rates. By analyzing traffic patterns and accident data, authorities can make data-driven decisions on where to implement road improvements or increase safety measures.
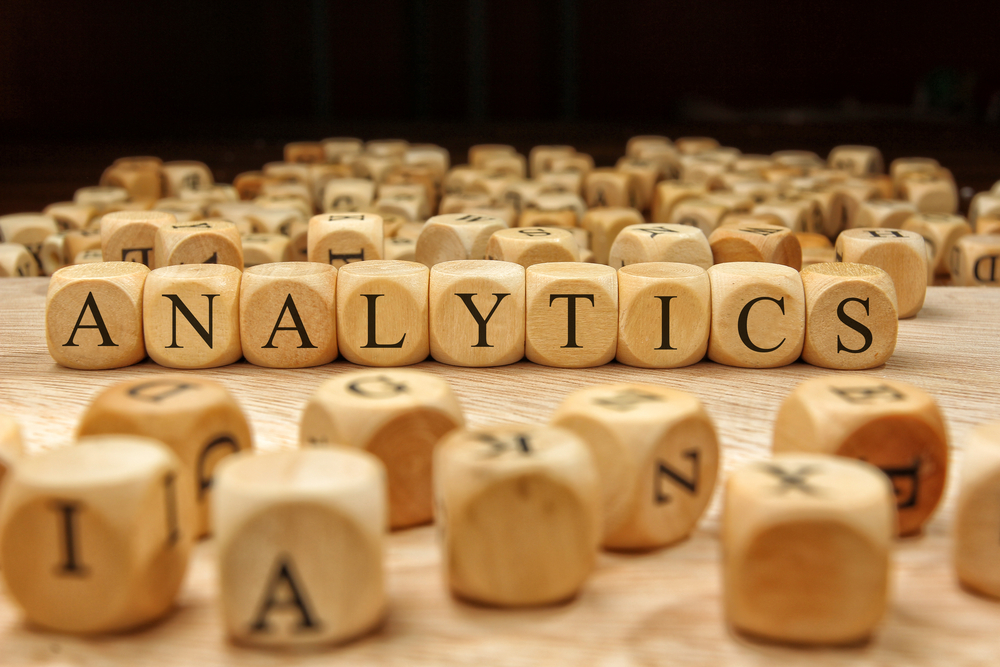
Benefits of Predictive Risk Analytics for Drivers
1. Enhanced Safety: By identifying potential risks before they happen, drivers can take preventive measures to avoid accidents. This leads to fewer road incidents and greater overall safety.
2. Cost Savings: For both insurance companies and drivers, predictive risk analytics can lead to cost savings. Drivers who engage in safer driving behaviors may see lower premiums, while insurers can better predict risk and reduce claim payouts.
3. Personalized Insurance Plans: Traditional insurance models often rely on broad factors to determine premiums, but predictive analytics allows for a more individualized approach. Usage based insurance models can lead to more accurate pricing based on actual driving behavior.
4. Proactive Maintenance: Predictive models can alert drivers about potential vehicle issues before they lead to a breakdown or accident. By addressing these issues early, drivers can avoid expensive repairs and dangerous situations.
5. Improved Decision-Making: Whether you’re a driver or a fleet manager, predictive risk analytics empowers you to make smarter decisions based on data rather than relying on intuition. From choosing safer routes to adjusting driving habits, the insights provided can significantly reduce risks.
Challenges to Overcome
While predictive risk analytics offer a wealth of potential benefits, there are still challenges to address:
• Data Privacy and Security: The collection and analysis of personal driving data raise concerns about privacy. Striking a balance between data utilization and protection is crucial.
• Accuracy of Models: Predictive analytics relies on high-quality data. Poor data quality or incomplete information can lead to inaccurate predictions, potentially increasing risk instead of reducing it.
• Driver Trust: Some drivers may be skeptical about the idea of being monitored, especially when it comes to insurance companies or fleet managers using driving data to set premiums or make decisions.
What The Future Holds
As technology continues to evolve, the potential of predictive risk analytics for drivers will only grow. We can expect more advanced systems that combine AI, real-time data, and IoT devices to improve road safety even further. From reducing insurance costs to saving lives, predictive risk analytics represents a transformative step toward a safer, smarter future on the roads.
In conclusion, predictive risk analytics is revolutionizing the way we understand and manage driving risks. By leveraging the power of data, we can make driving safer, more affordable, and ultimately save lives. Whether you’re an individual driver, an insurer, or a fleet manager, embracing these technologies will help you stay ahead of the curve in road safety.